Neuromorphic computing, the next generation of AI, will be smaller, faster, and more efficient than the human brain.
March 31, 2025
Download a print-friendly version of this article.
Artificial intelligence loves electricity. By one estimate, the cost to power the world’s large language models (LLMs) will surpass the gross domestic product of the United States by 2027. That’s an estimated annual electricity bill of 25 trillion dollars, and while that’s probably an overestimate, the point is: current AI is an energy hog. To counter this, researchers at Los Alamos and across the Department of Energy (DOE) are trying to develop AI that can operate on just 20 watts—the same amount of electricity that powers two LED lightbulbs for 24 hours and roughly the amount of energy consumed by the human brain each day. If successful, the approach is a pathway to AI that’s scalable and mirrors the adaptability of natural intelligence. “We could make a mosquito-sized drone as smart as a mosquito,” says Garrett Kenyon, a computational neurologist at the Lab who specializes in AI. Kenyon calls this still-experimental idea the next generation of AI.
At the core of this AI evolution is neuromorphic computing, a paradigm inspired by the human brain’s structure and function. Unlike current AI models, which rely on binary supercomputers to process billions or even trillions of parameters, neuromorphic systems use energy-efficient electrical and photonic networks modeled after biological neural networks. In Kenyon’s words, these networks use physical hardware to mimic nature’s most efficient and powerful inference and prediction engine. “Human brains can change based on their interpretation of the world,” says Kenyon. “AI, currently, can’t.”
To illustrate this shortcoming of LLMs, one that Kenyon hopes neuromorphic AI can help solve, he references a group of technological pranksters that recently tricked self-driving cars. They flashed the cars with t-shirts emblazoned with STOP signs. The cars, unable to discern context, responded by stopping, demonstrating the deterministic nature of current AI algorithms. The car’s behavior is a product of feed-forward processing. “I see a STOP sign, therefore I stop.” Neuromorphic computers, like biological networks, are designed to process information through feedback loops and context-driven checks. “I see a STOP sign. But that STOP sign is on a t-shirt. I drive on—cheeky kid.”
“The next wave of AI will be a marriage of physics and neuroscience,” says Kenyon. In September, he helped run the DOE’s 2024 Neuromorphic Computing for Science Workshop to prioritize which projects should receive funding through the 2021CHIPS and Science Act, which allocated 280 billion dollars toward, among other things, reestablishing American dominance in computing. At the conference, neuromorphic computing experts from around the country tackled questions such as: Should scientists start by reverse engineering an insect brain or a mouse brain? Which emerging low-energy microprocessors, like the memristors being developed now at the Lab’s Center for Integrated Nanotechnologies, are future systems most likely to use? Can existing supercomputers be used to better model neuromorphic computers before significant resources are committed to building them?
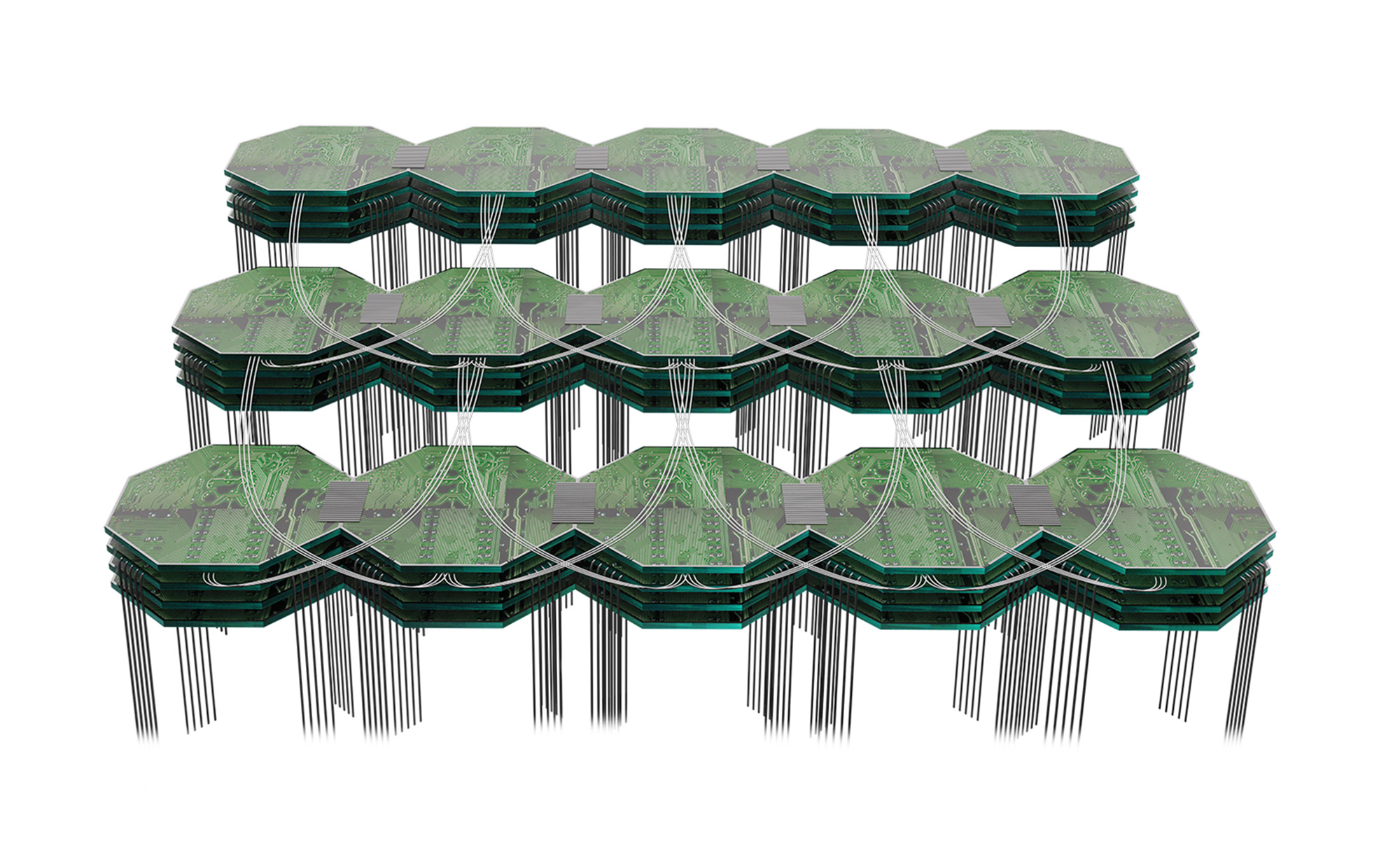
Currently, large neuromorphic computers feature just over a billion neurons connected by a bit more than 100 billion synaptic connections. The human brain has 100 trillion synaptic connections. But Kenyon and his collaborators at institutions including the University of Michigan and Pacific Northwest National Laboratory view these relatively small machines as proof that neuromorphic computing can be scaled to brain-level complexity. “Once we’re able to implement the full process of creating these networks in a commercial foundry, we can scale to very large systems quickly,” says Jeff Shainline, a collaborator of Kenyon’s who works at the National Institute of Standards and Technology. In other words, once you’ve fabricated one neuron, it’s not that much harder to fabricate a million. The scientists’ near-term goal is to build out a design for a neuromorphic computer that sits within a two square meter box and houses as many neurons as the human cerebral cortex. Calculations suggest this computer could operate between 250,000–1,000,000 times faster than a biological brain and use just 10 kilowatts of power, a bit more than a home air conditioning unit.
Kenyon believes this work is laying a path toward an advanced version of neuromorphic AI, one that consumes far less energy than current AI and behaves much more like the intelligence that’s trying to design it.
People also ask
- What is neuromorphic computing? A nascent and promising branch of computing, neuromorphic computing aims to mimic the structure and function of the human brain. Powered by highly efficient artificial synapses and neurons, like the roughly virus-sized memristor circuits developed at the Los Alamos-based Center for Integrated Nanotechnology, a neuromorphic approach could deliver a future where computers consume as much energy as the human brain—and are every bit as powerful.
- How does neuromorphic computing work? Neuromorphic computers are based on the biology of the brain, which in humans is packed with 100 billion neurons. Though the most advanced computers are still just a fraction of the human brain’s complexity, they work in a similar way, with timed electrical signals used to process information in networks of artificial neurons.