Lab scientists are using AI to guide the design of criticality experiments.
March 31, 2025
Download a print-friendly version of this article.
Predictive simulation is one of the things that Los Alamos does best. It is a primary tool for studying nuclear reactors, nuclear weapons, astrophysics, criticality safety, and more. These simulations of the most dynamic processes in the universe go hand in hand with experimentation: Experiments produce the data that go into the simulations, and the simulations guide the design of subsequent experiments—so the data used in simulations must be as high quality as possible. A recent project at Los Alamos, called EUCLID (Experiments Underpinned by Computational Learning for Improvements in nuclear Data), used machine learning (ML) and artificial intelligence (AI) to get high-quality data faster for some of the Lab’s most vital missions.
Criticality experiments on plutonium are done at the National Criticality Experiments Research Center (NCERC), a Department of Energy facility in Nevada. These elaborate experiments involve bringing plutonium to the point of criticality, the state in which a fission chain reaction is self-sustaining, with the number of neutrons generated being equal to the number lost. The reaction is self-sustaining but not uncontrolled. “It’s not like a nuclear weapon; there’s no yield,” assures Jesson Hutchinson, a Los Alamos nuclear engineer who led the EUCLID project. “It’s more like a mini reactor, so low in power that we don’t even need a cooling system.”
Broadly, the terms ML and AI are becoming less interchangeable, with ML trending toward automated pattern recognition and AI trending toward large-language models that mimic human intelligence. EUCLID used both: first, ML to identify nuclear data in need of improvement, then, AI to design an experiment to produce the improved data. The end goal of EUCLID and several related projects is to provide the Lab with two new ML/AI-based, mission-focused capabilities: a tool to reduce errors in nuclear data and a new way to design criticality experiments.
Nuclear data are used by simulations to predict what reactions will happen under various conditions. “Data from different experiments are combined into one big data set called a library,” explains Denise Neudecker, a Los Alamos nuclear physicist in charge of the nuclear data work for EUCLID. “So you can model things like weapons, reactors, and astrophysics and ask, ‘What will happen if I shoot this with that?’ and the model will pull data from the library and predict how many neutrons will be produced and whether it will be subcritical, critical, or supercritical.”
But nuclear data libraries don’t come straight from experiments; they are processed through a pipeline that combines experimental data with nuclear theory. Once processed, the data must be validated through further experiments before being made available to researchers worldwide.
This process has several shortcomings that EUCLID aimed to remedy. First, libraries aren’t as transferable as they could be because data is manually tuned to specific experiments, and the hand-tuning done for one experiment can become errors for a different experiment. Second, because of its complexity, criticality experiment design is iterative and time-consuming. Finally, the process is designed and evaluated by human brains, so it’s inefficient, and new nuclear data libraries are only released about every 10 years, meaning when new questions come up, the answers may be based on old information.
“We wanted to purposely look for places to use AI where the brain can’t go or is overloaded with information,” says Neudecker. “AI is not a magic wand; it’s a tool that can find trends in data that the human brain cannot.”
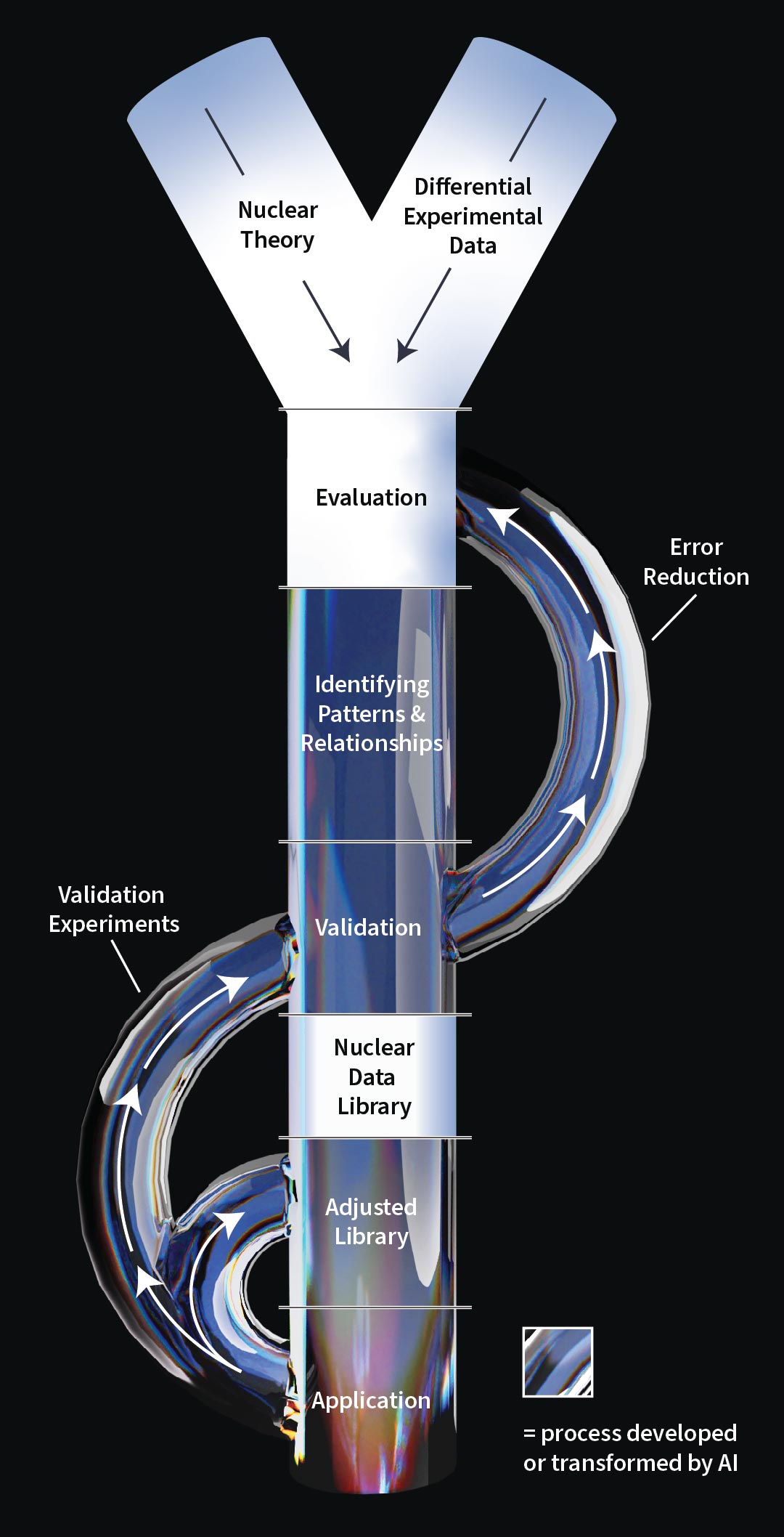
“Different libraries have different uncertainties, and two libraries can give the same answer to one question and different answers to another,” adds Hutchinson. “Those are the kinds of errors we want to reduce.”
EUCLID used simulation and experimentation to show that key segments of the nuclear data pipeline can be automated and enhanced. It was a crucial proof of concept: By reducing errors, there is less iteration, so the validation experiments match predictions sooner. The time to produce a new library could be brought down from 10 years to just three years.
“We used ML and AI in two ways,” says Mike Grosskopf, who led the ML/AI aspect of the project. “First, to identify sources of error, and second, to design an experiment that would improve the data.”
For the error-finding part of EUCLID, the scientists were interested in whether nuclear data were related, and how. They used ML and ML-interpretability methods to identify relationships and patterns in the data. ML interpretability has to do with human understanding of what the ML model did and why.
Grosskopf explains, “The model would find a pattern, and we’d ask the nuclear data experts, ‘Why are these things related? Is there reason to believe that a problem in nuclear data is causing a bias or are they related through other means?’ Then we’d iterate, removing known red herrings or focusing on a subset of data and putting it back through the ML model.”
The end goal is to provide the Lab with two new AI-based, mission-focused capabilities.
To test their idea, the team wanted to identify one error-prone thing to try and fix. ML indicated that fast nuclear data for plutonium-239 (239Pu) was something that criticality experiments were sensitive to. These are data from processes including fission and scattering of a 239Pu nucleus upon absorbing a neutron, so the team decided to use that as their target for error reduction.
For the experimental design part of EUCLID, AI was used to design a criticality experiment that would reduce errors in the fast nuclear data for 239Pu. Through a sequential approach known as Bayesian optimization—an AI model would evaluate a set of particle transport simulations for criticality and propose a design, then the scientists would run a new simulation, reupdate the model, and have it propose a modified design—the team eventually arrived at the design for a new criticality experiment, which was conducted in early 2023 at NCERC.
The experiment consisted of two configurations of 239Pu, one to maximize neutron leakage and one to minimize it. For each configuration, two subcritical masses of 239Pu were brought into proximity with one another via remote control until criticality was achieved. Over the next 10 minutes, neutrons streamed silently into detectors, carrying with them key information about the systems’ criticality.
The team used the collected data for new particle transport simulations and calculated the uncertainty. The data were analyzed by Neudecker and other experts in the loop, then adjusted and assessed as to whether there were significantly fewer errors. There were. But the data also revealed previously unknown details about 239Pu scattering that are now being looked at more closely, including an overestimation of more than 10 percent for one variable.
EUCLID was preceded by a project that focused on using AI for experiment optimization and followed by an ongoing project focused on improving nuclear data for tantalum, an important metal used in plutonium manufacturing.
Another follow-on project, led by Neudecker, is PARADIGM (PARallel Approach of Differential and InteGral Measurements). “Whereas EUCLID was focused on criticality experiments, PARADIGM is focused on speeding up the entire pipeline, especially for intermediate energy ranges, which are the least well understood,” she explains. PARADIGM will bring Los Alamos and NCERC experiments together, but the novelty is how the experiments will be selected: The team is using AI methods to understand which combinations of theory and experiment will best reduce uncertainty in nuclear data.
We really want to use AI in places where the human brain can’t go.
EUCLID involved a team of roughly 50 people, including nuclear data experts, AI/ML experts, criticality experts, theorists, and engineers. It also included a training element, with the Department of Energy’s Nuclear Criticality Safety Program, a key sponsor of EUCLID, using the project to provide invaluable training to new students and scientists.
“A big part of this success comes from linking together people from these different communities,” says Hutchinson. “That helped us with EUCLID and continues to be a boon for other projects moving forward.”
People Also Ask:
- What is nuclear data? Nuclear data is information about the structures and interactions of atomic nuclei. Scientists rely on nuclear data for nuclear energy production (from both fission and fusion), space exploration, nuclear non-proliferation, and nuclear medicine.
- What are criticality experiments? Criticality experiments are highly controlled experiments that bring a fissile material like plutonium or enriched uranium to the point criticality, when the number of neutrons being generated is equal to the number being lost.