Los Alamos scientists are accelerating vaccine and drug development by combining experimental data and AI.
March 31, 2025
Download a print-friendly version of this article.
As soon as COVID-19 began sweeping across the globe, people everywhere started to talk about antibodies. Did we already have antibodies from some past illness? When would a vaccine be available to help us make our own antibodies? Could we get antibody therapy from a recovered patient?
The global response to COVID-19 was propelled by the fact that the virus, SARS-CoV-2, is closely related to the SARS coronavirus that emerged in 2003. Building on prior knowledge about that original SARS virus, and armed with 17 years worth of research since, scientists quickly designed and produced vaccines to prevent SARS-CoV-2 infection and drugs to combat the disease it causes—both deeply rooted to the role of antibodies that specifically recognize this novel pathogen.
“What if we don’t have 17 years of research to help us respond to the next threat?” asks Los Alamos biologist Jessica Kubicek-Sutherland.
Vaccine and drug development is famously slow—oftentimes taking a decade of research, testing options one at a time, before clinical trials even begin. The response to COVID-19 was a stark reminder that the research and development process is not agile enough to respond quickly if something truly new comes along. Furthermore, SARS-CoV-2 mutated, as viruses often do, leading scientists back to the labs to make molecular updates. Scientists and decision makers began to set their sights on reducing the time it takes to develop new vaccines and therapeutics. Could they dramatically shrink the 10-year timeline? What could be acheived in just one year or less?
Los Alamos scientists are key players in two separate projects aimed to give the world a more nimble posture ahead of the next biothreat by enabling a quick response with a high degree of confidence. The vaccine-development project RAPTER and the drug-development project GUIDE are both using artificial intelligence (AI) and experimental studies to cut through the slow slog of traditional research methods and bring a laser-like focus to the processes of vaccine and drug development.
By coupling predictive AI and targeted experimental work, Los Alamos scientists are answering the question: If humanity is faced with a looming pandemic, how can decision makers quickly decide where to put their resources for the best outcomes?
Quick path to prevention
There are many different approaches to making a vaccine, but they all follow the same broad strokes: An antigen, which is a pathogen or just a piece of one, stimulates the host’s immune system to produce antibodies. Antibodies are protective proteins that recognize and bind to the antigen to generate an immune response. In addition, the immune system creates “memory” cells that can make antibodies in the future to help prevent additional infections by that pathogen. There are many different vaccine platforms, or ways of packaging the antigen and presenting it to the host immune system, each with its own pros and cons. There are also multiple different molecules from a given pathogen that could serve as antigens. Testing every antigen from every pathogen on every platform can be impractically time consuming, but knowing which combinations work and which don’t is vital.
Los Alamos is the lead institution for RAPTER (Rapid Assessment of Platform Technologies to Expedite Response), a program funded by the Defense Threat Reduction Agency of the Department of Defense to accelerate vaccine development. RAPTER is a collaboration among 14 institutions that is using AI together with lab experiments to create a predictive tool to help decision makers find the vaccine sweet spot— the best combination of vaccine platform, pathogen, and antigen—for any known threat and likely any emerging one.
Choosing the right antigen is critical. Some vaccines, like flu vaccines, use the whole pathogen as an antigen, after either killing it or weakening it substantially. These vaccines take a long time to develop because extensive tests are needed to ensure efficacy and safety. Some vaccines, like a popular chicken pox vaccine, use a subunit—a protein or sugar molecule found on the outside of the pathogen—as an antigen. Many newer vaccines use, instead of the entire organism or subunit, a small portion of genetic information that encodes instructions for the host’s body to make its own antigen-like molecules. For example, the SARS-CoV-2 virus has characteristic spike proteins covering its surface. The spikes help the virus infect a host’s cells, and small areas of the spikes serve as antigens for our immune systems. Most COVID-19 vaccines deliver only the genetic instructions for these small portions of the spike protein; the host’s own cells then use those instructions to manufacture antigens, launching an immune response. Vaccine platforms can use different vectors, such as mRNA or adenoviruses, to deliver the instructions. (Adenoviruses normally cause mild colds but can be modified to instead carry antigen-making instructions).
Vaccine and drug development is famously slow—oftentimes taking a decade of research.
As well as whole-organism, subunit, and vector-based platforms, completely novel vaccine approaches are also being explored. One such approach uses nanosponges, synthetic particles disguised to look like cells, that entice the pathogen to infect them instead of infecting the host’s real cells. Some vaccine platforms are better suited for certain pathogens and not others, and some platforms have been extensively studied while others are more nascent. The research literature base is huge and varied, making it hard to know which studies have been done, what they showed, and where the gaps in knowledge lay.
“Our main goal is to more thoroughly understand what each vaccine platform is capable of and what organisms they are best used for,” says Kubicek-Sutherland, principal investigator for the RAPTER program.
Ultimately, RAPTER will provide a predictive tool that can take any pathogen’s genetic sequence data and instantly, systematically lay out the best options for vaccine development to facilitate a rapid response to a disease outbreak. To achieve this goal, the nearly 100-scientist RAPTER team is building the tool using three components: knowledge extraction with AI and machine learning, mechanistic modeling to inform the AI, and in vitro and in vivo experiments to test promising candidates in real biological systems. The team is building the RAPTER tool from the ground up, starting with three pathogens: SARS-CoV-2, ebolavirus, and Burkholderia pseudomallei, the bacterium that causes a disease called melioidosis, which is considered a significant biological hazard.
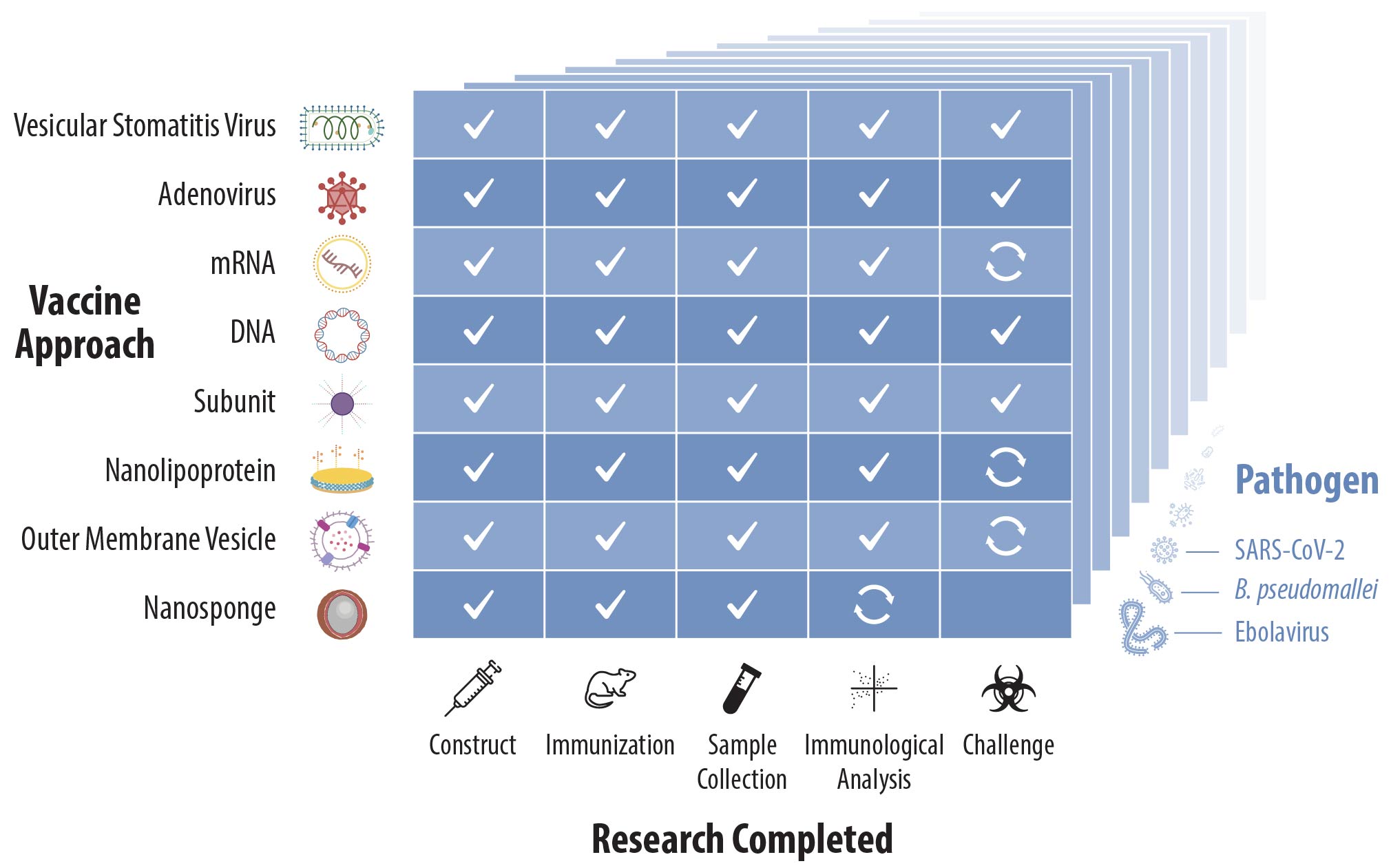
RAPTER partners at Pacific Northwest National Laboratory and Northeastern University developed an AI language model to evaluate scientific literature and government databases (e.g. Food and Drug Administration [FDA], Centers for Disease Control and Prevention) and create an inventory of existing vaccine research about the three target pathogens; this is the knowledge extraction step. The model gathers key information, like which antigen was used in a vaccine experiment, the dosing schedule implemented, and the immune response observed, as well as the efficacy rating and any possible clinical trials that are planned or complete. AI is extremely helpful in speeding up the work, as there are over 300,000 scientific publications with the word “vaccine” in the abstract. But the scientists need more than a keyword search; they need the AI to determine which papers have useful data and to extract those data.
“The language model was originally developed to understand cancer pathways, so we’ve been adapting and curating it for vaccine-related terminology. It’s taken a lot of back-and-forth with data scientists and experts,” says Kubicek-Sutherland. At first, the tool could only identify about 1 in 10 papers that were relevant to the RAPTER project, but now it’s more like 7 out of 10. The team is also cross-validating sources and trying to ensure that the AI is using data that were collected in a standardized way, ensuring the best results. In addition to training the tools to properly use vaccine-related words, the Los Alamos partners are teaching it to understand human immunity, step by step.
“At Los Alamos, we have a lot of experience learning how vaccines work through computational immunology. We have spent over 30 years creating mechanistic models to mimic the body’s immune response,” says computational biologist and RAPTER co-investigator Benjamin McMahon. “Using these tools, we have modeled every single step of the immune system process, from its general attack on a new threat to the rapid evolution of highly precise antibodies and memory cells that provide lasting protection.” Vaccines need to work in a similar way: They must effectively provoke the immune system to evolve the best possible antibodies now and to produce them again in the future.
McMahon and the RAPTER team at Los Alamos are using their mechanistic models to make data training sets on the immunity process. The training sets help the AI learn to better identify patterns in the immune response from the literature data. The mechanistic models also help the scientists with immunobridging, a process by which they look at the data available—which might be limited—and try to extrapolate it to something new. For instance, can they take data from an ebola vaccine trial in mice plus data from humans who have recovered from ebolavirus infections and use both to predict how a vaccine might perform in humans?
The third part of the RAPTER program is testing in biological systems, which can be anything from culturing human cells in a dish to doing experiments with animals. Based on AI-assisted analyses, the scientists identify areas where more data are necessary. For instance, the AI indicated that only two vaccine platforms have been tested with Burkholderia pseudomallei. As a result, RAPTER partners at the U.S. Army Medical Research Institute of Infectious Diseases (USAMRIID) are now conducting mouse studies using the other six platforms so that a thorough comparison can be included in the RAPTER tool.
“These experimental data are critical to ensure the accuracy of the RAPTER tool,” says Los Alamos biologist Katie Davis-Anderson. In addition to experimental data from partner institutions such as USAMRIID, Kubicek-Sutherland and her team, including Davis-Anderson, at Los Alamos are setting up in vitro immunization assays using human cells to further close the gap between animal and human data.
Fast-tracked treatment
Antibodies can also be given, ready made, to a person to prevent or treat disease if their own immune response is inadequate. Although vaccination shows the body an antigen to provoke an immune response, giving premade antibodies to bolster or speed up that response is called immunotherapy. Immunotherapy can be especially helpful for individuals, such as cancer patients, whose immune systems are weak or compromised: If they cannot effectively produce their own antibodies, immunotherapy provides antibodies to help.
In 2021, an antibody-based drug called Evusheld was approved for use in immunocompromised patients to help prevent COVID-19 infections. Evusheld antibodies were designed to attach to SARS-CoV-2 spike proteins in a way that blocked the virus from binding to and infecting host cells.
Unfortunately—although not surprisingly—SARS-CoV-2 mutated (and continues to mutate). Mutations in the virus’s genetic code can translate into tiny adjustments to the shape of the antigen. When this happens, antibodies might still attach, but not as strongly, diminishing their effectiveness. In late January 2023, the emergency use authorization for Evusheld was revoked because the drug was no longer effective enough against the newly-emerged Omicron variant.
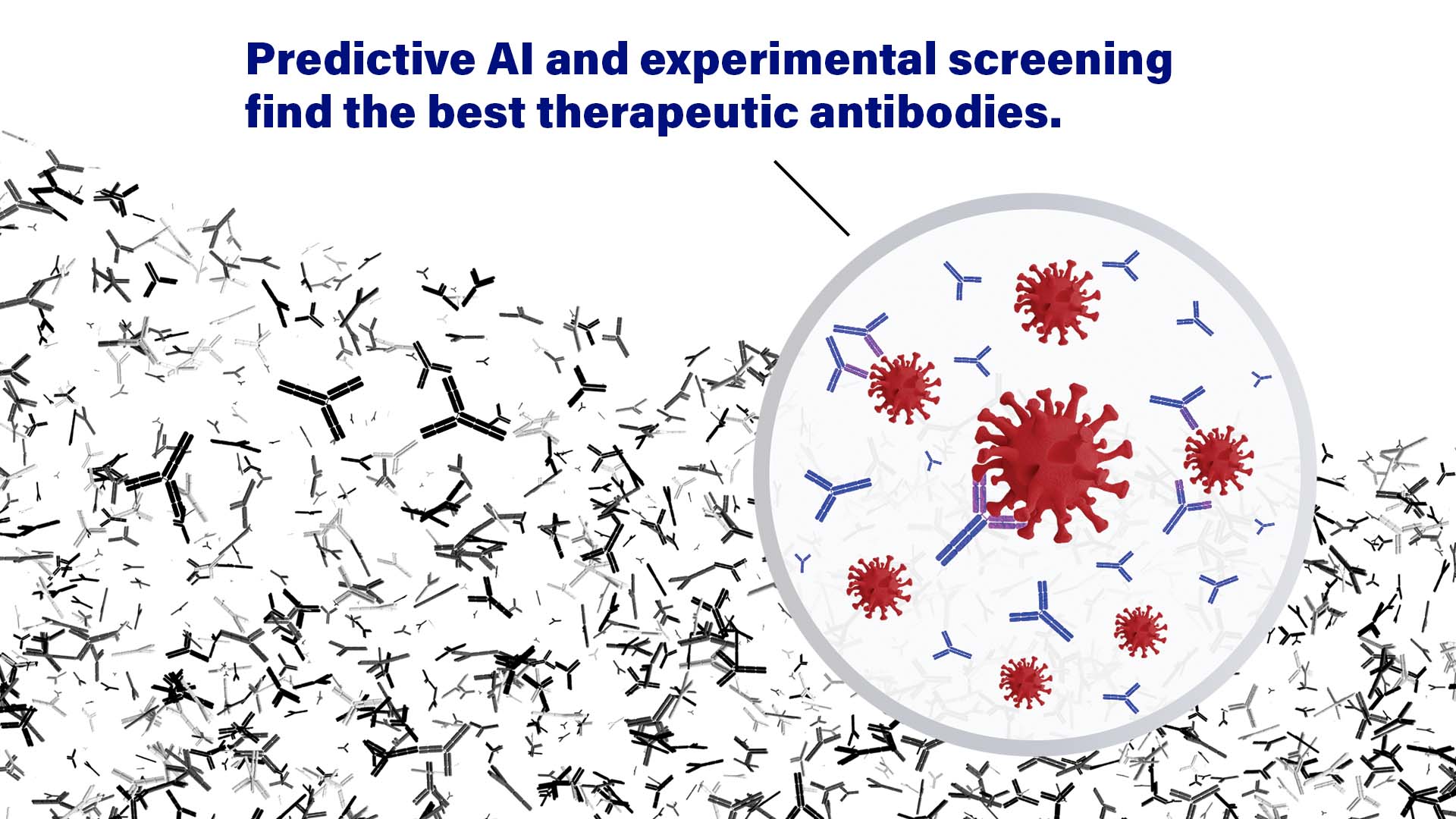
Los Alamos biologist Antonietta Lillo is a partner in a drug development project called GUIDE (Generative Unconstrained Intelligent Drug Engineering) that is using AI to accelerate development of all types of antibody-based immunotherapies. GUIDE is funded by the Joint Program Executive Office for Chemical, Biological, Radiological and Nuclear Defense on behalf of the Department of Defense. The question GUIDE aims to answer is: Can computational methods, including simulations, as well as AI, accelerate the development of therapeutic antibodies?
The goal of GUIDE is to create a pipeline to fast-track new therapeutic antibodies through the research phase and to accelerate FDA approval. By integrating AI and experimental antibody screening, the 10 GUIDE partner institutions are working on a rapid process to reprogram therapeutic antibodies into safe and manufacturable drugs for any new threat. The collaboration hopes to ultimately collapse the drug development process from nearly a decade to 120 days or less.
When antibodies are produced naturally, each generation is better adapted to the pathogen than the previous generation. This process leads to a large spectrum of antibodies, each with a unique binding affinity that is encoded genetically. The GUIDE team takes advantage of this wide spectrum of antibody diversity, knowing that each change to the genetic code potentially leads to important improvements to antibody binding. The team starts with the genetic codes for antibodies that are known to be manufacturable, nontoxic, and capable of recognizing multiple variants of a given pathogen family. The computational scientists then use AI to optimize the properties of the antibody’s binding region to make it the best possible match to a specific pathogen. For one of their first projects, the GUIDE partners started with the genetic code for antibodies used in Evusheld: Could the team design new antibodies that would be effective against the Omicron variant of SARS-CoV-2?
To tackle this multi-objective problem, the AI team used three different protein structure tools to create new candidate antibodies for SARS-CoV-2 strains and to predict their binding affinity. The algorithms sought to improve binding to the new Omicron strains while simultaneously maintaining affinity to older, but still circulating, strains such as Delta. The team also used a predictive tool to assess thermal stability and a large language model with deep learning to assess the viability of the candidates for use in humans. The team used an iterative process, a so-called “optimization loop,” to quickly search the huge set of possible antibody sequences with the goal of finding candidate antibodies that would bind strongly, be nontoxic to humans, and be easily manufactured. The team’s computational process explored a design space of 1017 possible antibody sequences, executing 168,000 binding simulations and ultimately selecting 376 high-confidence designs and a further 359 lower-confidence designs.
Next, Los Alamos stepped in to assess and verify candidate antibodies. These candidates were still virtual—each existing only as a string of DNA instructions selected by AI. To be sure that the antibodies would bind as expected, experimentalists needed to translate each DNA sequence into an actual antibody molecule, allow it to bind to a SARS-CoV-2 antigen, and then evaluate its binding efficacy and other attributes. Typically, this process of producing hundreds of individual antibodies and testing them one by one is tedious and prohibitively time-consuming and expensive. Fortunately, Lillo and the Los Alamos team have a time-tested method to speed up the screening by evaluating all the candidates en masse.
For over two decades, Los Alamos scientists have specialized in a high-throughput antibody development and screening capability that uses a technique called yeast display. The process uses yeast cells as little factories, each producing one kind of antibody and displaying copies of it on the cell’s surface. The scientists wash the cells with fluorescently labelled antigens (such as SARS-CoV-2 spike proteins), allowing them to bind to any antibody that has the right fit. Key to the process is that each yeast cell retains the DNA that encodes its displayed antibody, so that when an antibody-antigen match is made, the team can easily isolate the appropriate yeast cell and identify the corresponding DNA code.
Lillo and her colleagues screened 458 of the candidates, including 99 high-confidence choices and the further 359 aggressive, low-confidence designs, to ensure that highly fit sequences were not being overlooked by the computational methods. They also screened for manufacturability and toxicity in parallel. In the end, the Los Alamos scientists validated eight top AI-chosen candidates, confirming that those antibodies could in fact be used for new drugs. They also found four additional candidates, including a long-shot winner.
“To our surprise, we found an antibody that outperformed the top candidate among high-confidence sequences. This discovery shows the value of pairing AI and experimental screening, ” says Lillo.
Now that they have finished redesigning antibodies for a SARS-CoV-2 therapeutic, the current focus of the Los Alamos GUIDE scientists is to generate data about several virus families to help improve the GUIDE AI capability overall. Using yeast display to screen millions of antibodies, the scientists are gathering insight about which genetic sequences correspond to binding specificity, thermostability, and toxicity, all of which will help train the AI about those particular virus families. These comprehensive data sets that identify both good and bad therapeutic candidates will be used to help the AI tools learn what makes an antibody thermostable and nontoxic. “The more experimental data we have, the better AI works,” says Nick Generous, a Los Alamos biologist on the GUIDE project. “In fact, it won’t help to only train AI with polished, published data; we need to train AI on good and bad data so that it can tell the difference.”
The future is now
Of all the ways AI could impact life on earth, facilitating rapid response to disease threats is one of the most positive. Infectious diseases pose a threat to all organisms—humans, animals, and plants. Our changing climate, lifestyles, agricultural practices, and interwoven global societies together create more opportunities for disease emergence and spread, so as soon as we conquer one threat, another could be on the doorstep. AI is not magic, but its potential for revolutionizing the length of time it takes to make a new vaccine or drug does seem like wizardry. And by using Los Alamos’ experimental and computational expertise to both train and complement AI, our scientists are honing their superpowers.
People also ask
- How are new drugs made? Drug development is a several step process that can take years, if not decades. It involves selecting a target associated with a disease and systematically testing therapeutic compounds that interact with it. Predictive AI, experimental studies, and mechanistic models can be used revolutionize the speed of this process.
- How are vaccines created? By studying and mimicking immunology, scientists make vaccines using various techniques to stimulate a person’s immune response. The vaccine concept then undergoes several rounds of testing for efficacy and safety, which can take years to complete.